At the end of November, PANCAIM team members Natalia Alves and Megan Schuurmans, PhD students at Radboud UMC, presented insights from their research in the project at RSNA in Chicago, the leading global radiology conference.
As a unique and ambitious project aimed to significantly improve Pancreatic cancer diagnosis, prognosis and the general patient pathway, PANCAIM members know the challenges but also the great potential of exploiting already available data for the development of AI for healthcare settings. Gathering, exchanging and annotating relevant data, identifying relevant biomarkers and other features, developing AI models, and ensuring clinical validation to enable responsible use in the hospital – it is not an easy task but the potential benefit for clinicians and patient is considerable.
See the abstract below for an outline of their research. Thanks to Megan and Natalia for giving PANCAIM a stage, and lots of success for continuing their research!
“Towards Safe Clinical Use of Artificial Intelligence for Cancer Detection Through Uncertainty Quantification” – Natália Alves
In order for AI to be safely integrated in the clinic, AI can be used to predict uncertainty and identify uncertain cases with lower performance that should be handled with extra care. Therefore, the goal of this research is to investigate whether quantifying deep learning (DL) models’ uncertainty can help identify low performance cases that require expert attention. This study included two use cases:
- pancreatic cancer detection on contrast-enhanced computed tomography
- and clinically significant prostate cancer detection on biparametric magnetic resonance imaging.
15 models were trained with the nnUNet framework and integrated into previously established pipelines for each use case. The models were applied independently to the test sets and uncertainty was quantified in a case level as the standard deviation of the ensemble. The DL frameworks’ performances for the uncertain groups were significantly lower than for the certain groups for both use cases. To conclude, the proposed ensembling method can be used to identify cases where AI models’ predictions are uncertain and show low performance.
“Deep Learning for Detection of Iso-attenuating Pancreatic Adenocarcinoma in Computed Tomography” – Megan Schuurmans
Iso-attenuating pancreatic cancer (pCa) is linked to earlier stages of disease and better outcome, but is challenging to detect on CT. Therefore, the goal of this research is to investigate the ability of deep learning to localize iso-attenuating pCa in computed tomography (CT) images and assess the effect of including prior anatomy information on localization performance. This study included contrast-enhanced CT scans in the portal venous phase of 44 patients, who had a visually iso-attenuating tumour.
Two previously developed deep learning (DL) algorithms based on the 3D nnUnet framework were applied to the set of iso-attenuating lesions: one considering only tumour information for training (nnUnet_T) and one considering surrounding anatomical structures (nnUNet_MS). The average precision for the nnUNet_MS was 80.03 % ± 8.6%, while for the nnUnet_T model it was 72.87% ± 7.1% (p<0.05). This indicates that surrounding anatomy aids the localization of iso-attenuating pCa lesions. To conclude, the proposed 3D nnUnet_MS model can be used to detect and localize iso-attenuating lesions.
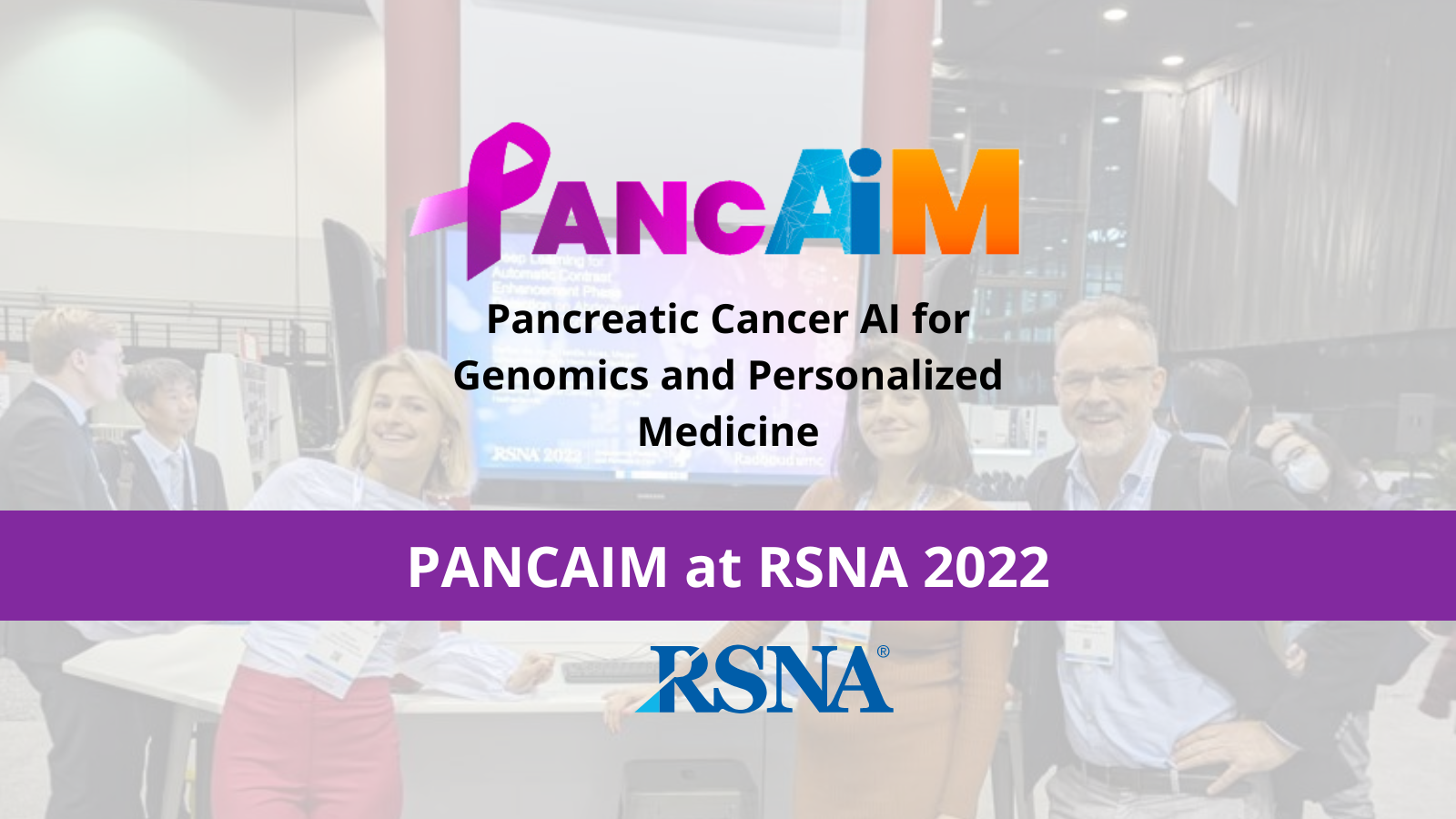