Pancreatic ductal adenocarcinoma (PDAC) is one of the deadliest cancers worldwide, associated with a 98% loss of life expectancy. Image-based artificial intelligence (AI) can help improve outcomes for PDAC given that current clinical guidelines are non-uniform and lack evidence-based consensus. However, research on image-based AI for PDAC is too scattered and lacking in sufficient quality to be incorporated into clinical workflows.
Our international and multi-disciplinary PANCAIM team at Radboud University Medical Center, some of the world’s leading experts in pancreatic cancer, has prepared a review article “Setting the Research Agenda for Clinical Artificial Intelligence in Pancreatic Adenocarcinoma Imaging” which has recently been published in the Journal Cancers.
The article breaks down the patient pathway and pinpointing the current clinical touchpoints in each stage and analyses available PDAC imaging AI literature addressing each pathway stage. Then, current performance and pitfalls are identified in a comprehensive overview. Finally, the article proposes a future research agenda for clinically relevant, image-driven AI in PDAC.
Read the full article open-access here!
Authors
Megan Schuurmans, Natália Alves, Pierpaolo Vendittelli, Henkjan Huisman, John Hermans
Abstract
Pancreatic ductal adenocarcinoma (PDAC), estimated to become the second leading cause of cancer deaths in western societies by 2030, was flagged as a neglected cancer by the European Commission and the United States Congress. Due to lack of investment in research and development, combined with a complex and aggressive tumour biology, PDAC overall survival has not significantly improved the past decades. Cross-sectional imaging and histopathology play a crucial role throughout the patient pathway. However, current clinical guidelines for diagnostic workup, patient stratification, treatment response assessment, and follow-up are non-uniform and lack evidence-based consensus. Artificial Intelligence (AI) can leverage multimodal data to improve patient outcomes, but PDAC AI research is too scattered and lacking in quality to be incorporated into clinical workflows. This review describes the patient pathway and derives touchpoints for image-based AI research in collaboration with a multi-disciplinary, multi-institutional expert panel. The literature exploring AI to address these touchpoints is thoroughly retrieved and analysed to identify the existing trends and knowledge gaps. The results show the absence of multi-institutional, well-curated datasets, an essential building block for robust AI applications. Furthermore, most research is unimodal, does not use state-of-the-art AI techniques, and lacks reliable ground truth. Based on this, the future research agenda for clinically relevant, image-driven AI in PDAC is proposed.
Keywords:
Pancreatic cancer; artificial intelligence; imaging; radiology; pathology
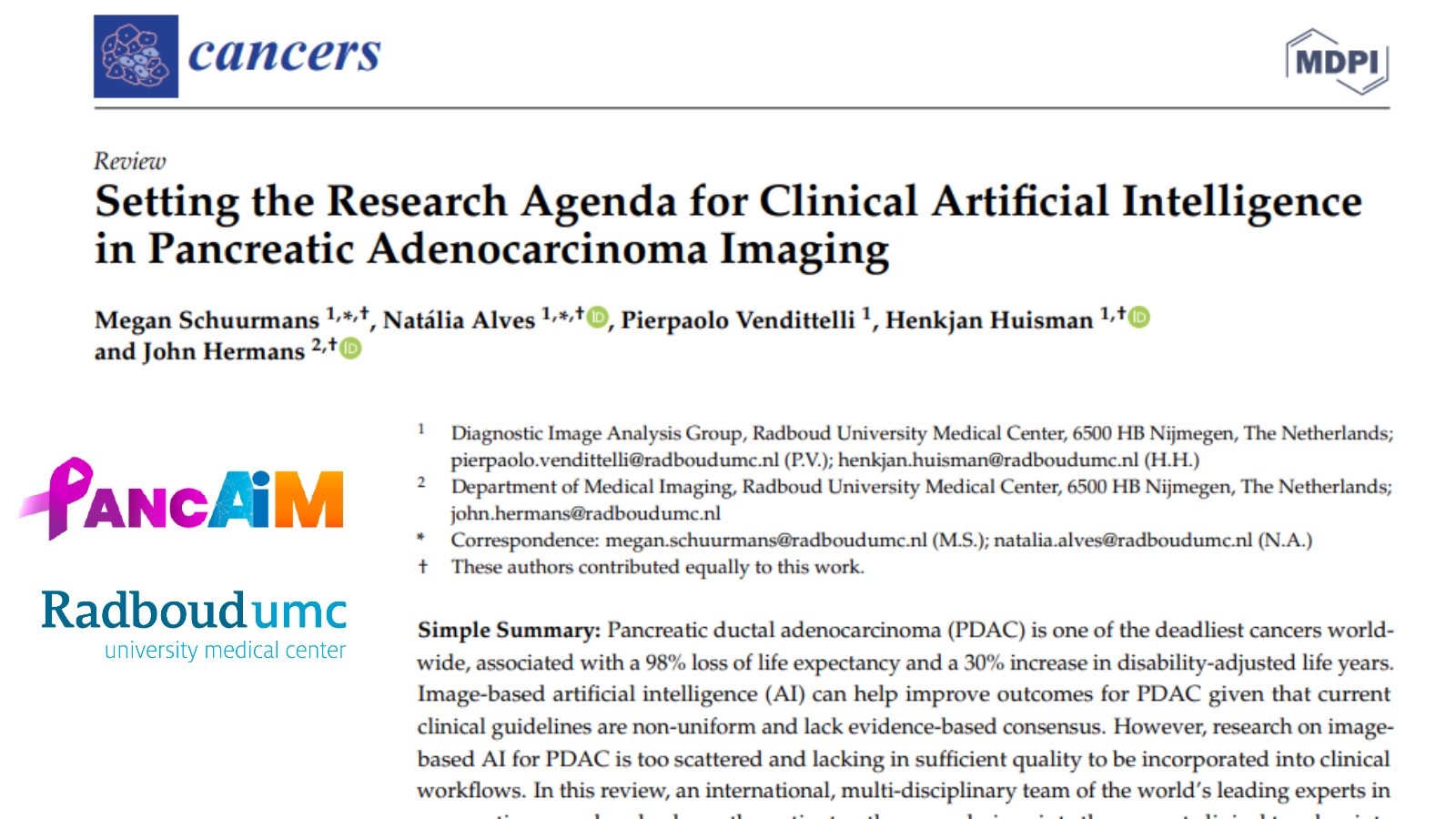