We are happy to share that are PANCAIM team members Natália Alves, Henkjan Huisman, John J. Hermans from project coordinator Radboud UMC, along with their collaborators have published a new article title “Prediction Variability to Identify Reduced AI Performance in Cancer Diagnosis at MRI and CT” in the prestigious radiology journal!
We know that advancements in Artificial Intelligence (AI) hold immense promise for transforming cancer diagnostics worldwide and alleviate the global cancer burden by assisting clinicians and radiologists in decision making. But there’s a critical gap that needs addressing: While much of the medical AI research focuses on algorithm development, there’s a pressing need to also ensure their safe integration into individual patient care. One key challenge in this lies in understanding & communicating the uncertainties associated with AI predictions: AI should not only make accurate predictions but also acknowledge when it doesn’t have the answer. This would make the integration of AI into clinical practices significantly safer and foster trust among clinicians.
This problem inspired PANCAIM researchers to explore Uncertainty Quantification (UQ) in AI. Their study focused on evaluating AI prediction variability as a practical metric for uncertainty for identifying cases at AI-failure risk in cancer diagnosis at MRI and CT across different cancer types, data sets, and algorithms. Their study considered three separate clinical tasks in the retrospective analysis of multicenter data sets: detection of pancreatic ductal adenocarcinoma (PDAC), detection of clinically significant prostate cancer (csPCa), and estimation of pulmonary nodule malignancy risk. For each clinical task, a publicly available AI algorithm that did not originally include UQ was expanded to produce an uncertainty score associated with its predictions.
The results are encouraging: Researchers found that AI led to significant accuracy improvements in the “certain” group, where AI accuracy was comparable to clinicians – however, AI performance much worse when UQ classified the results as “uncertain”. Using AI to triage “uncertain” cases produced overall accuracy improvements for pancreatic and prostate cancer (+5%) and lung nodule malignancy prediction (+6%) compared to a no-triage scenario. These findings present a framework within which AI can be implemented more safely by alerting clinicians to cases in which AI’s analysis might fall short – and enabling humans to step in and pick up the slack.
Check out the full article here: https://pubs.rsna.org/doi/10.1148/radiol.230275
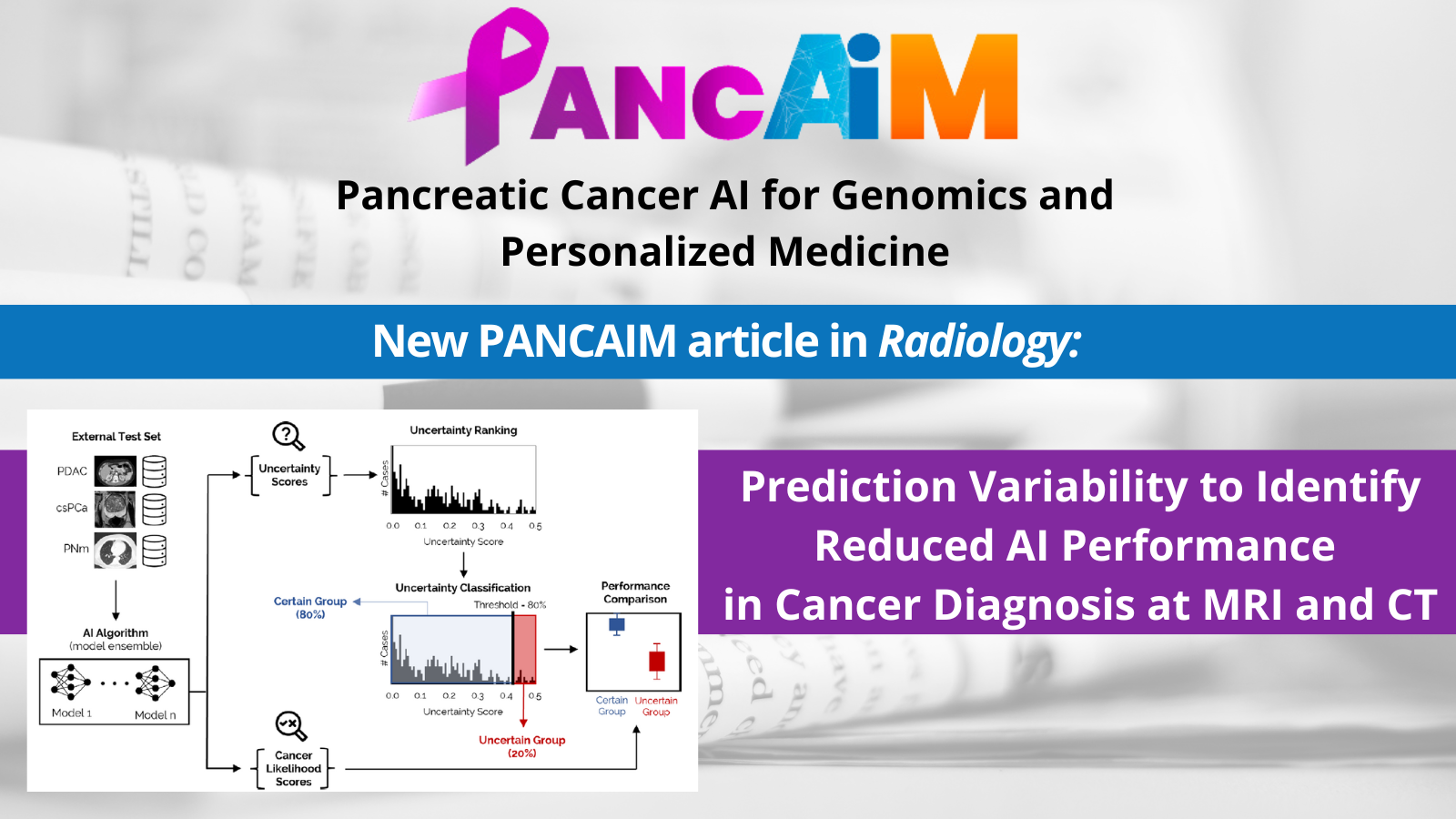